Artificial Intelligence (AI) is transforming cities by optimizing transportation, improving public safety, and enhancing sustainability. AI-powered technologies are making urban areas more efficient, reducing traffic congestion, and improving energy management. In this article, we will explore how AI is shaping the future of smart cities.
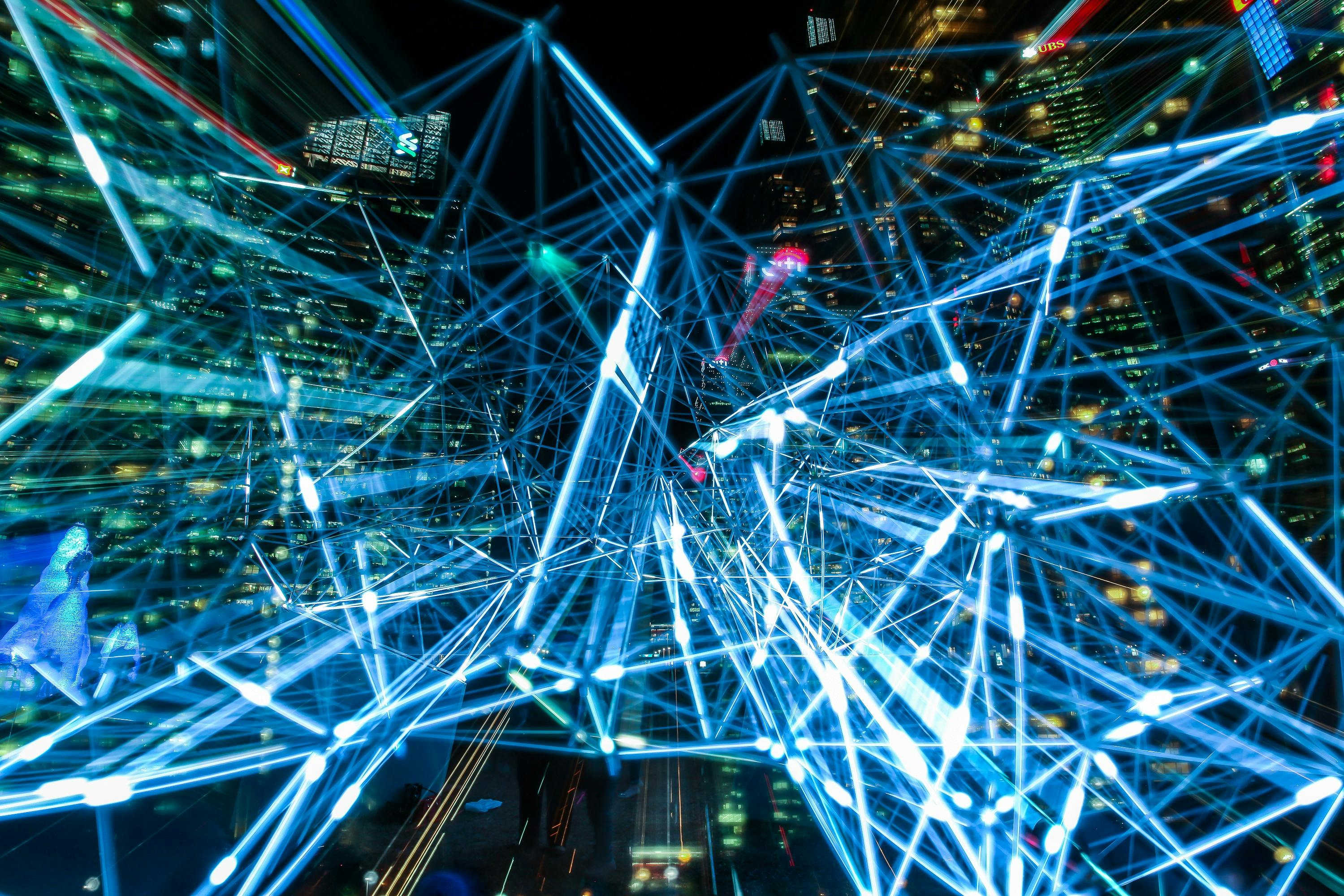
1. The Role of AI in Smart Cities
AI enhances urban life by:
- Managing traffic flow to reduce congestion. AI-powered traffic management systems analyze data from thousands of sensors, cameras, and connected vehicles to dynamically adjust traffic signals, optimize routing, and predict congestion before it occurs.
- Enhancing security through AI-powered surveillance. Advanced computer vision and behavioral analysis algorithms monitor public spaces, detect unusual activities, and alert authorities to potential security threats while respecting privacy regulations.
- Optimizing energy consumption for sustainability. Smart grid technologies use machine learning to balance energy demand and supply in real-time, integrate renewable energy sources, and reduce waste in municipal power systems.
The integration of AI across urban infrastructure marks a significant evolution from isolated “smart” technologies to interconnected systems that communicate and coordinate with each other. This holistic approach allows cities to address complex challenges that span multiple domains, such as the relationship between transportation patterns and air quality.
Urban Impact: According to the Smart Cities World Report (2024), cities that have implemented comprehensive AI-powered traffic management systems have seen average commute times decrease by 15-25%, while reducing carbon emissions from vehicles by up to 30% in central business districts.
2. AI Applications in Smart Cities
A. AI in Transportation
AI-powered traffic lights adjust based on real-time congestion data. These intelligent systems use computer vision and predictive analytics to monitor traffic flow and pedestrian patterns, dynamically adjusting signal timing to prioritize congested routes, public transportation, or emergency vehicles as needed.
Autonomous public transport systems reduce emissions and increase efficiency. Self-driving buses, trams, and shuttles optimize routes based on demand patterns, operate at consistent fuel-efficient speeds, and can be deployed strategically during peak hours without the limitations of driver schedules.
“Smart transportation isn’t just about reducing congestion—it’s about reimagining mobility as an integrated service rather than a collection of separate modes. AI gives us the ability to orchestrate entire transportation ecosystems, from buses and trains to shared vehicles and micromobility options, optimizing the entire network rather than individual components.”
— Dr. Elena Rodriguez, Urban Mobility Researcher
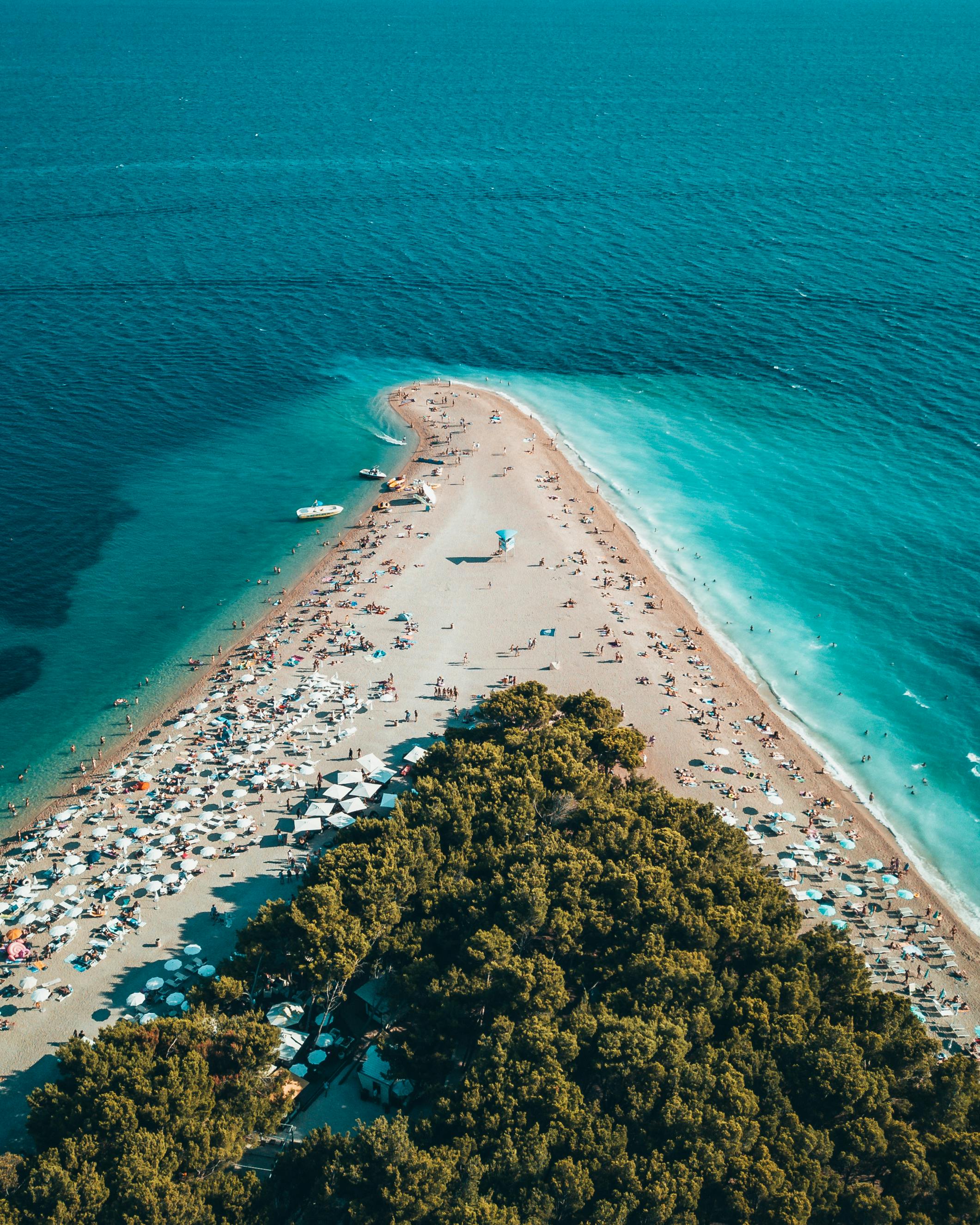
B. AI in Public Safety and Security
Facial recognition cameras help detect criminal activity. These systems can identify persons of interest in crowded public spaces, supporting law enforcement in locating missing persons or tracking suspects. However, their deployment is increasingly governed by privacy regulations and ethical guidelines to prevent misuse.
AI-driven emergency response systems predict disasters and optimize response times. Machine learning algorithms analyze data from weather stations, seismic sensors, flood monitors, and other sources to provide early warnings for natural disasters. During emergencies, AI systems can optimize the deployment of first responders based on incident locations, traffic conditions, and resource availability.
Urban security expert Carlos Santos explains: “The most effective smart city safety systems don’t just react to incidents—they prevent them. By analyzing patterns from thousands of data points across a city, AI can identify potentially dangerous situations before they escalate. For example, we’ve seen systems that can predict street flooding based on rainfall patterns and drain capacity, allowing city workers to clear critical drains before major storms.”
C. AI in Energy Management
Smart grids optimize electricity distribution and reduce power waste. AI algorithms predict energy demand patterns, balance loads across the network, integrate intermittent renewable energy sources, and identify potential outages before they occur. These intelligent grid systems can also isolate failures to prevent cascading blackouts across urban areas.
AI-powered buildings adjust heating, cooling, and lighting based on occupancy. Advanced building management systems use sensor networks and machine learning to maintain optimal comfort conditions while minimizing energy consumption. The AI can predict occupancy patterns, account for weather conditions, and even learn individual preferences in different zones of large buildings.
Efficiency Benchmark: The International Energy Agency reports that commercial buildings equipped with AI-powered energy management systems reduce electricity consumption by an average of 25-35% compared to conventional buildings of similar size and purpose. In large urban centers, this translates to significant reduction in carbon emissions and infrastructure strain during peak demand periods.
3. AI and Sustainability in Cities
Artificial intelligence is powering sustainable urban development through several key applications:
- AI helps cities monitor air quality and predict pollution levels. Networks of IoT sensors feed data to machine learning models that can identify pollution sources, predict air quality issues based on weather patterns and traffic conditions, and recommend interventions like temporary traffic restrictions or public transit incentives on high-risk days.
- Waste management AI improves recycling and waste collection efficiency. Smart waste systems use computer vision to sort recyclables more accurately than manual methods, while AI-optimized collection routes reduce fuel consumption and vehicle emissions. Some advanced systems even use predictive analytics to schedule collections based on container fill levels rather than fixed schedules.
- AI-driven climate control systems reduce energy consumption in urban areas. District heating and cooling networks use machine learning to predict thermal energy demand and optimize production and distribution, while urban heat island mitigation strategies employ AI to identify priority areas for green infrastructure development.
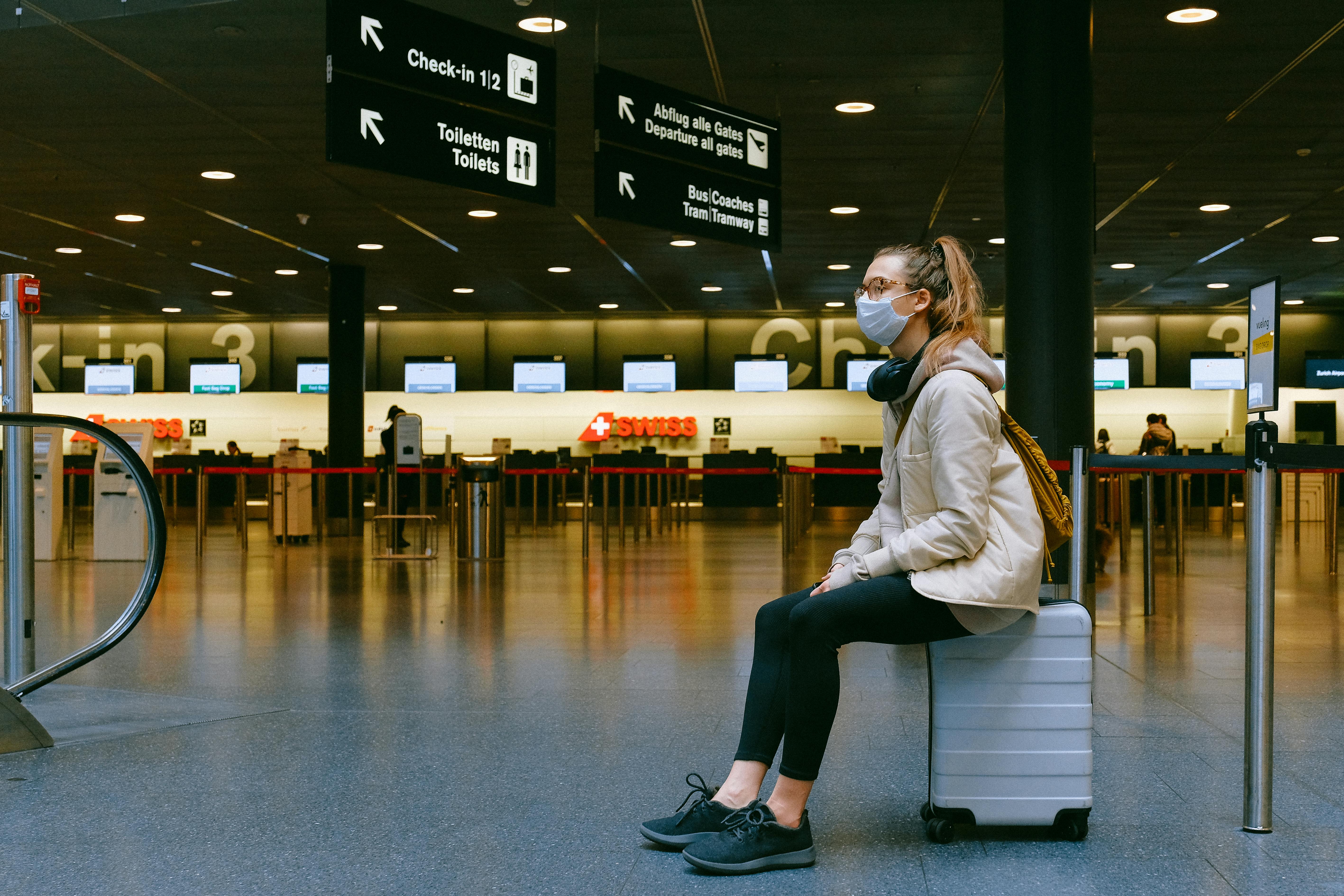
Environmental technology specialist Dr. Maria Chen notes: “What makes AI particularly valuable for urban sustainability is its ability to find patterns and relationships that human analysts might miss. For example, an AI system in Barcelona identified correlations between specific traffic patterns, weather conditions, and elevated particulate matter that led to targeted interventions reducing pollution exposure in sensitive areas by nearly 20%. This kind of nuanced approach simply wasn’t possible before we had the computing power and algorithms to process massive environmental datasets.”
4. The Future of AI in Smart Cities
As artificial intelligence and urban technologies continue to evolve, several emerging trends will shape tomorrow’s smart cities:
- AI-powered self-driving taxis for efficient urban mobility. Autonomous vehicle fleets will optimize routes collectively rather than individually, reducing empty vehicle miles, congestion, and the need for parking infrastructure. These systems will seamlessly integrate with public transportation to provide comprehensive mobility solutions.
- AI-enhanced disaster prediction models to prevent natural catastrophes. Advanced simulations combining historical data, real-time monitoring, and physics-based models will provide increasingly accurate predictions of floods, wildfires, and other natural hazards, enabling more effective preventive measures and evacuation planning.
- AI-driven smart city planning for optimized infrastructure development. Urban planning tools will use AI to simulate countless development scenarios, predicting impacts on traffic, housing affordability, public health, and environmental quality to guide policy decisions and infrastructure investments toward optimal outcomes.
“The next generation of smart cities will be characterized by what we call ‘anticipatory governance’—using AI not just to react to problems more efficiently, but to identify and address potential issues before they manifest. This approach requires integration across traditionally siloed government departments and a willingness to trust data-driven insights, even when they challenge established practices.”
— Professor James Li, Director of Urban Innovation Lab
Emerging Research: The Urban AI Initiative at MIT is developing “digital twin” technology that creates complete virtual replicas of cities, allowing officials to test policy interventions and infrastructure changes in a simulated environment before implementing them in the real world. Early pilots of this technology have helped identify unforeseen consequences of proposed transit changes and optimize the placement of new public facilities.
Conclusion
AI is revolutionizing urban life by making cities smarter, safer, and more sustainable. From transportation to public safety and energy management, AI-powered technologies are shaping the future of smart cities.
The evolution toward truly intelligent urban environments represents one of the most promising applications of artificial intelligence, with the potential to dramatically improve quality of life while reducing environmental impact. As these technologies mature and become more widely adopted, we can expect cities to become increasingly responsive to the needs of their residents, adapting in real-time to changing conditions and preferences.
Embrace AI-driven urban innovation and experience the city of the future!
References and Further Reading
- Smart Cities World. (2024). Global Implementation Impact Report: AI-Powered Traffic Management Systems. Smart Cities World Research Division.
- Rodriguez, E., & Patel, S. (2024). Integrated Mobility as a Service: AI Coordination of Urban Transportation Networks. Journal of Intelligent Transportation Systems, 28(2), 187-204.
- Santos, C., & Ivanova, M. (2023). Preventative Urban Security: AI-Driven Approaches to Public Safety. International Journal of Smart City Applications, 14(4), 312-329.
- International Energy Agency. (2024). Energy Efficiency in Smart Buildings: AI Control Systems Analysis. IEA Technology Report 2024-05.
- Chen, M., & Williams, T. (2024). Pattern Recognition in Urban Environmental Data: Case Studies in Air Quality Management. Environmental Science & Technology, 58(3), 1542-1559.
- European Commission Urban Development Division. (2024). Waste Management Optimization Through AI: Comparative Study of European Cities. EU Urban Sustainability Report.
- Li, J., & Garcia, A. (2024). Anticipatory Governance in Smart Urban Planning. Cities: The International Journal of Urban Policy and Planning, 136, 103997.
- Massachusetts Institute of Technology Urban AI Initiative. (2025). Digital Twin Cities: Simulation-Based Urban Decision Support Systems. MIT Urban Studies and Planning Technical Report.